

Additionally, extensive experiments are conducted on three remote sensing image datasets with simulated and real noisy labels to quantitatively evaluate the performance of ICCE. Besides, the error bound of ICCE is derived with strict mathematical proof under different types of noisy labels, which ensures the advantages of ICCE from theory. The ICCE improves the robustness of DCNN to noisy labels by revisiting the sample weighting scheme so that much attention is paid to the clean samples instead of the noisy samples. Lets dig a little deep into how we convert the output of our CNN into probability - Softmax and the loss measure to guide our optimization - Cross Entropy. Then, an improved categorical cross-entropy (ICCE) is proposed to address the issue of noisy labels. These are tasks where an example can only belong to one out of. def addsampleweights (image, label): The weights for each class, with the constraint that: sum (classweights) 1.0 classweights tf. They use the following to add a 'second mask' (containing the weights for each class of the mask image) to the dataset.
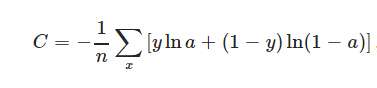
To solve this problem, the negative effect of noisy labels on remote sensing image classification by DCNNs is analyzed. Categorical crossentropy is a loss function that is used in multi-class classification tasks. I think this is the solution to weigh sparsecategoricalcrossentropy in Keras. However, noisy labels are often included in the training samples generated by VGI, which inevitably affects the performance of DCNNs. In recent years, volunteered geographic information (VGI) is widely used to train deep convolutional neural networks (DCNNs) for high-resolution remote sensing image classification.
